Análisis y comparación de modelos de clasificación de aprendizaje automático aplicado a riesgo crediticio
DOI:
https://doi.org/10.33017/RevECIPeru2017.0014/Keywords:
artificial intelligence, machine learning, credit risk, mathematic models, gradient boostingAbstract
The financial industry has become into a very competitive sector worldwide. In that sense, the credit granting decision is one of the most important process of all, and in whose accuracy, rests the good performance of several critical business KPI's such as loans level, credit recoveries level and nonperforming loans ratios. This key process has historically based on the experts’ judgement, and have taken the decision of granting or not credit loans according to several customer credit behavior elements. In the last decade, the developing of certain technology such AI and machine learning has allowed this process automation. The present paper has its main goal, the analysis of several mathematical algorithms based on machine learning and the exposition of which of them have the better results in credit granting predictions to collaborate with current knowledge in this particular issue, giving an objective explanation of the results and suggesting following researches to be developed in order to get better results in existing mathematical algorithms. As results of the experimentation determined that the best model was Gradient Boosting, with an accuracy of 83.71%.
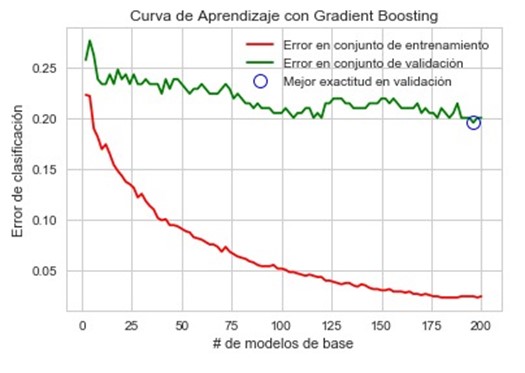